Introduction
In biology, the concept of interaction network is heavily used. Based on this concept, Network-based drug repositioning have been developed and can be grouped based on their main source of biological data: regulatory, metabolic and drug interaction networks.
Here, we will describe further the types of algorithms that can be encountered as network-based.
At Reckonect, we divided these algorithms into 2 different categories based on types data that was used: 1. The one using structural data of the drug or 2. algorithms using other type of data, like scientific articles or bioassays for example.
Description
Using the chemical structure
The structural data of a drug is the most essential information that could be used in order to find other targets or other mechanism of actions for repositioning purposes.
Using structural data gives the opportunity to discover new drug-target-interactions (DTI) based on structural similarities between the drug of interest and other drugs already working in the drug market. In order to do that, the models use âmolecular-input line-entry systemâ (or SMILES) strings and amino acid (AA) sequences, both 1D string inputs. This data, available in databases such as DrugBank or PubChem, would also rise the possibility to do molecular docking: simulation of drug-ligand binding based on the putative 3D structure of the drug.
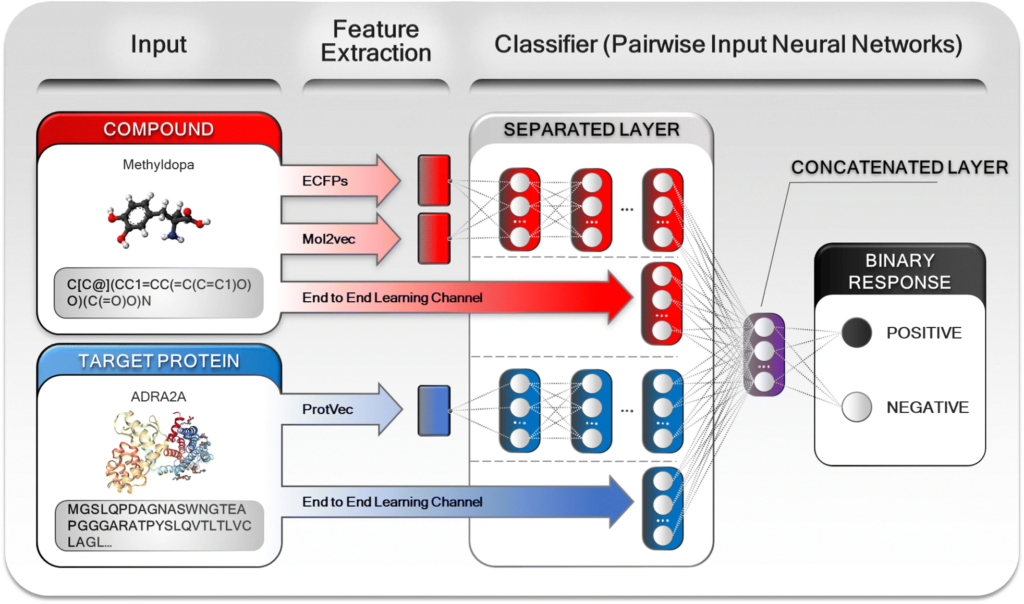
Using other type of data
Since 2020, new type of strategies emerged based on NLP algorithms or associated. The working hypothesis would state that by analyzing text-based data on a drug we could predict other type of possible interactions to ligand and therefore find new pathways on which the drug could have an effect.
Based on this idea, these algorithms could use for example:
- Bioassays (from available databases) to predict hit compounds for targets other than their originally intended purposes, (i.e. âassay repositioningâ). This approach does not rely on physicochemical features but solely on the bioactivity datasets of either the target or the ligand.
- From PubMed articles, using abstracts, PDFs, keywords and more in order to predict a drug repositioning based on other close drug data fitting the same pattern.
- Other types of data could be used as well but even more powerful, multi-modal data are now being used in order to repurpose drugs. For example Genome-wide Associated Studies (GWAS), Phenome-wide Associated Studies (PheWAS), protein-protein interactions (PPI) and other types of databases (like Pharos, Open Targets or STRING) are combined together to score priority targets that could be active with the drug.